
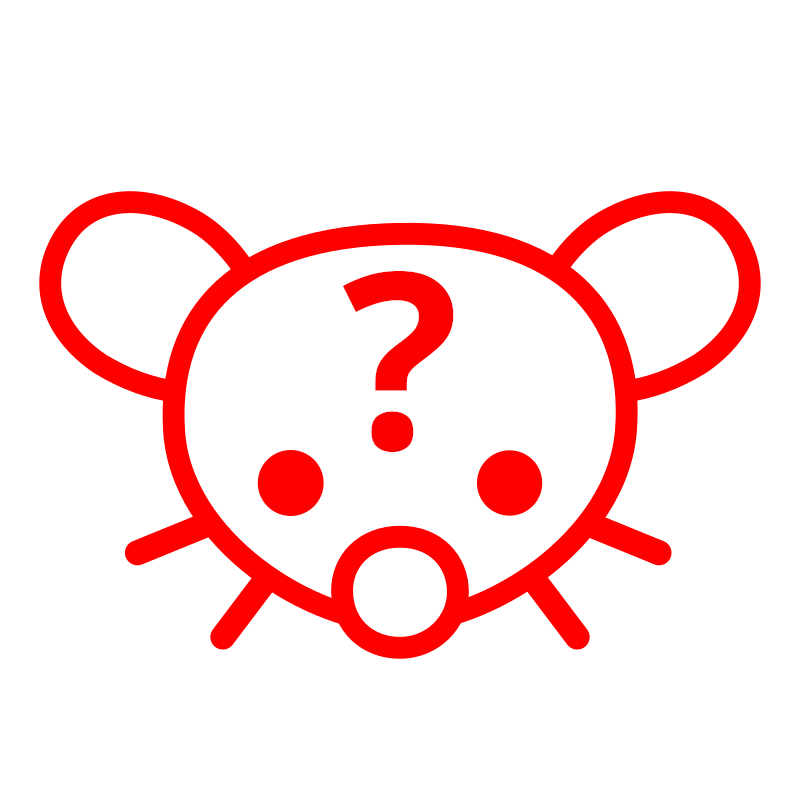
A sort of country blues song about how my cat would ignore my friend until they went into the bathroom to poop, and then the cat was all about hanging out and being affectionate.
“You only love me when I’m pooping…”
A sort of country blues song about how my cat would ignore my friend until they went into the bathroom to poop, and then the cat was all about hanging out and being affectionate.
“You only love me when I’m pooping…”
The funniest part is, the poorest people i know have a hatred for the poor.
I think people often hate things that remind them of things they don’t like about themselves
I had a job that paid stupid well. I’d give some money to almost anyone who asked on the street. They need it. I have plenty. I’m not going to notice the $1 or $5 or even $20 that I gave away. I make more than that in interest every day.
Rarely, I’ll talk with them a little. Ask their name or their story. There’s a regular around me that seems delighted that I remember his name.
Sometimes someone will seem unsafe, but most people are alright. It helps that I’m a bearded man, so certain classes of danger and harassment are rarely pointed at me.
I got laid off in February. I still give to people who ask, but I carry less cash so sometimes I don’t have anything to give.
When I’d be out with coworkers, before we all got laid off, none of them would give anything to people asking. I know they made as much or more than I did. I don’t judge people for not giving cash out when they’re in debt or struggling to pay rent, but I do judge my coworker for like wearing a high five figure watch and never helping the poor.
That management and leadership are smart, visionary, people without whom everything would fall apart.
It doesn’t matter what my line of work is. Management is mostly out of touch idiots everywhere.
“We need to redesign the web page to be more modern! Get me a big hero image and an image carousel!”
“Customers are complaining about how they can’t save their search settings. Maybe we should do something about that?”
“No that’s not a priority”
Outside of like NYC you have a car. It’s a bad system economically, ecologically, and socially, but many people are kind of stupid and reactionary. You show them how putting in a bike lane and adding a bus stop will lower car traffic, improve air quality, and increase economic activity and they just go “no because I feel so”. Or, “one time I had to move a refrigerator so we need to prioritize large privately owned vehicles”.
If more people had a backbone and spoke out / refused to implement shitty stuff, this wouldn’t happen.
Thus, it’s more than just the web developers 😄 . Product needs to have a backbone to stand up to their boss, too. I fought really hard to get rid of the mouse tunnels at that job, but was blocked by product and one of the directors of eng. It was mostly [office] political nonsense
Also, many design choices are entirely on the web developer.
Not at most of the companies I’ve worked out. There’s a design person or team. Eng can give feedback, but it’s pretty rare for them to be given a blank check.
I’m not gonna change my opinion until websites become usable again, you’re wasting your time on me.
That’s fine. Some web developers are morons, but some of everyone are morons. We can partially agree.
Most web developers are morons in the sense that most people are morons. They’re not especially moronic.
A lot of problems on the web aren’t coming from the developers. They’re management pushing for stuff.
Like, at an old company the UI had really bad mouse tunnels (mouse over menus and sub menus that close if you mouse out). Terrible interface. But someone in management liked it and no one would approve changing it. Easy to look at it and say we’re all morons, but most of the stupid there was from leadership.
Capitalism is a root cause. Let’s get rid of that, or if that’s too extreme that severely regulate it.
Jail all of Facebook’s decision makers. Seize it (investors get nothing), and either shut it down or revert it to a simple message board. Require moderators. Ban the trash (eg: sovereign citizens groups). Remember that time they tried to see if they could make people sad by changing the algorithm? Find those people and ruin them.
Pay labor more. Work them less. I’d just do basic income, personally.
Make more walkable spaces. Fuck car culture. You don’t meet anyone when you drive. Everywhere could have local spots where you see regulars.
More free public events. Brooklyn does “movies under the stars”. There’s also like yoga classes, bird watching, concerts. More of that.
Offer free education for anyone who applies in good faith. Offer classes on a range of subjects, but honestly I think a lot of people would benefit from lessons and practice on “how to talk to people” and public speaking.
Kind of a ramble. But I think if you leave capitalism in place, you’re going to have problems. “Everything has to make the owners as much money as possible, immediately” isn’t a formula for a good life.
I don’t know man, i think the simpler answer of “capitalism and profit motive create incentives that don’t yield good outcomes” is compelling. Okcupid was better and more feature rich before Match bought it.
There is some user error on the apps, though, as you say.
I would prefer basic, subtle, black. I don’t want rainbows and lights. I’m very function over form.
I’d also rather play a game on medium settings where it runs flawlessly and doesn’t make the fans go hard, than at high settings with worse performance.
Yeah I think people are too stupid on average to understand. Like how kids think pouring water from a short wide glass into a tall glass means there’s more water, people think one long line means longer wait times.
I feel like education could mitigate this but at least in the US our education isn’t a priority
Perhaps a universal icon for “pick language” would be helpful, like we have a icons for volume control and share. Good luck getting it adopted though.
For some reason getting a passport is like $200, plus whatever it takes to get the required supporting documents (eg: birth certificate, the photo). That’s not much by many metrics, but a lot of people in the US just don’t have $200 to spend.
Dislike:
I want it to get to a level where you find the good stuff reddit used to have. Like niche hobbies, detailed analysis, etc.
I’m doing my part, I guess
Possessive is normally done with an apostrophe. The dog’s toy. The apartment’s lobby.
But when possessive goes on the pronoun “it”? No apostrophe.
Look out for the monster. Its fangs are sharp.
If you put an apostrophe, that’s for “it is”.
This bothers me slightly. There’s history illinonating why it happened, but I don’t like it.
Larger orgs probably have their own website, and could put it there and/or make an RSS feed. That’s old tech, and probably not as popular because it can’t be monetized like twitter/facebook/etc.
There’s also email, which is typically free. It’s less fancy, but it’s also very open. You don’t get good discoverability, though. Some bands I like send out newsletters and that’s how I keep up with them.
Lastly, the fediverse exists. It has some of the problems of social media, but at least it’s not owned by a handful of rich assholes.
I don’t use reddit aside from reading search results. I never post and never explicitly seek it out.
I deleted Twitter years ago. I never used Facebook or Instagram or tiktok. That shit’s bad for you.
It’s annoying that some places will make announcements only on shitty platforms. None of my friends are this “hardcore” , so sometimes I find out about stuff like a concert or protest or whatever because one of them sees it on Instagram
CrossCode has such charm. The puzzles are good, but I find myself tired after playing it in a way other games don’t give me. I should finish it.
Shadowrun for Genesis was amazing! Ahead of its time. The way it semi randomly generated jobs for you to do was pretty unique. Like Bethesda radiant quests, but decades earlier and better. I really enjoyed rising up from the weakest street runner to someone with enough reputation to skip the line at the expensive club.
The leveling system was also pretty advanced for Genesis.
Also the cyberspace hacking was wacky and fun.
The main things reddit has going for it is more people, and better SEO.
Privately owned for profit orgs are extremely vulnerable to enshittification.